Analytical Marketing
Section 7–Step Five: Generate Insights with Analytics
By David Bartenwerfer
Just like the phrase Big Data, the practice of Analytics continues to gain more attention in board rooms. In the context of marketing, analytics promises to transform the way companies engage with customers. As companies get more savvy about employing data and analytics to make better decisions, having the ability to generate insights using complex mathematical methods will become an increasingly crucial point of competitive differentiation.
Marketing organizations can take full advantage of the benefits of data and analytics if they have laid the groundwork outlined in in previous sections. At this stage, they have thoughtfully considered their data and analytics strategy, are working toward procuring and managing that data, have made the investments to automate their processes, and developed the right metrics. The final piece of this puzzle is developing the capability to build advanced analytics models for predicting and optimizing outcomes.
Returning to the Big Data theme, organizations should understand that the real issue isn’t that your organization is acquiring large amounts of data, it’s what you’re doing with the data that matters. The best vision for marketing organizations is that they should be able to take data from any source, harness that data with analytics and find insights that enable cost or time reductions, increased rates of customer acquisition, reduced customer attrition, optimized new product development offerings, and in general, smarter business decisions.
The Approach to Modeling
How to build those predictive or optimization models? The most effective approach to taking a more scientific view of an operation is to borrow elements of the scientific method — creating a hypothesis regarding the relationships between key variables and then seeking the information to prove or disprove that hypothesis.
Analytical models that have the greatest impact usually start with identifying a business opportunity and determining how an analytical model can improve performance. This hypothesis-led approach generates faster insights and bases models in real life relationships between data that are better understood by marketers.
When companies are considering the role of analytics in their enterprise, recall that there are four main categories of analytics: business intelligence, descriptive analytics, predictive analytics, and prescriptive analytics (or optimization). As a refresher, here are the descriptions of each:
- Business Intelligence:reporting about what is happening, usually in real-time or over a defined time period. Examples: sales pipeline by product by geography; the response rate for a marketing campaign; new customer acquisitions by month; or net promoter score.
- Descriptive analytics: an analysis of historical data, the conventional reports and intelligent dashboards that business leaders use to better understand their business. Examples: buyer segmentation and their traits; how high value customers differ from the average and what defines those customers.
- Predictive analytics: use of past and present data with the application of statistical models to predict (or score) what may happen in the future. Examples: who will respond to this marketing campaign; what are the potential values of each prospect in the lead database; or who will defect and when.
- Prescriptive analytics (or optimization): the insights from “what if” models that tell you what to do and determines new ways to operate. Examples: what if we allocate more resources to brand building instead of call-to-action campaigns; what would be the most optimal combination of marketing spend to maximize ROI; which new product features and pricing are likely to maximize revenue.
Exhibit 1 shows the continuum of possibilities for analytical tools from the basic reports to the more comprehensive and complicated analytical models such as optimization and risk adjusted optimization.
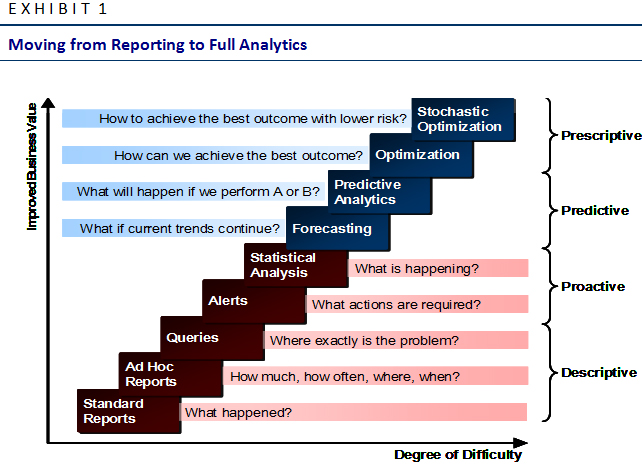
difference between predictive analytics and forecasting as both disciplines look to the future. However, forecasting is the process of making predictions based on past and present data and an analysis of trends and does so on an aggregate basis — seeking to predict the values of whole populations. Predictive analytics seeks to predict the behavior at an individual level.
As mentioned previously, because data and technology will fuel analytics, it is important for marketers to identify their current status on that front and what type of analytics they will require in order to be a more competitive and better positioned organization in this age of digital disruption. A clear vision of the desired business impact must shape the approach to defining data needs, analytical tools/frameworks and any required organizational change.
Models drive Metrics
Once marketing organizations have built their marketing data mart and performed some elemental analyses, they can start building new metrics around those insights. While senior execs are mostly interested in revenue and volume numbers, marketers should focus on understanding how their programs are driving gains in those metrics. Exhibit 2 shares several examples of new metrics that can be considered using the entire suite of analytical options from the descriptive to the prescriptive.
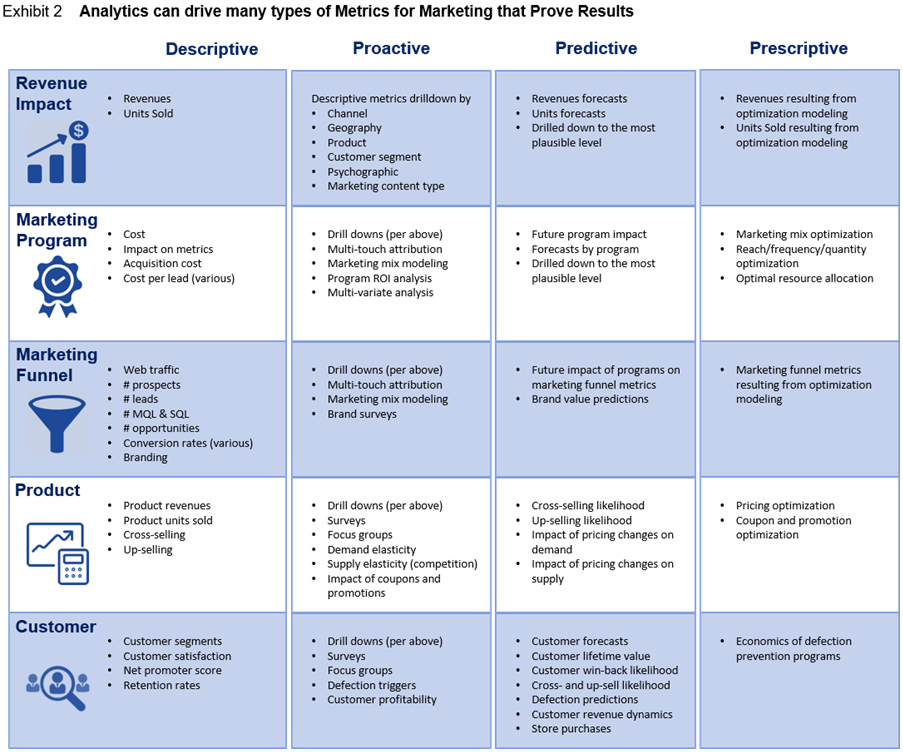
The two main forms of advanced analytics are predictive and prescriptive analytics. The following three chapters will demonstrate how to use each to improve the marketing function.
Author
David Bartenwerfer is the founder and principal of Quantum Consulting and Technology. QuantumCT helps product and marketing organizations get smarter and prove, predict and optimize impact and ROI with economic and financial modeling that employs customizable algorithms and technology leading to fast and lasting insight and action. Mr. Bartenwerfer has over twenty years’ experience in the High Tech, Internet, Telecom, Media, Financial Services and Retail industries and holds a B.S. in Systems Engineering with minors in applied mathematics and economics from the University of Virginia and an M.B.A. from the Stanford Graduate School of Business. For further information, contact the author at davidbartenwerfer@quantumct.com.